Probability
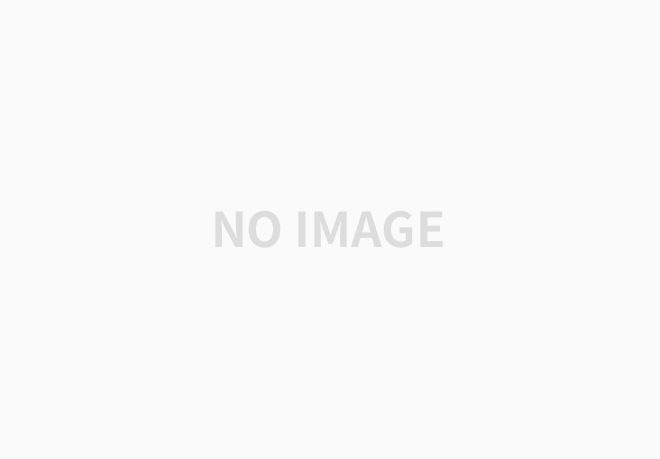
어떤 확률 분포 (distribution = theta) 가 존재할 때,
특정 범위에서의 확률 P( x1 <= X <= x2 | theta ) 이라고 볼 수 있다. (area under curve)
즉, 고정된 특정 distribution에서의 확률값이라고 이해할 수 있다.
Likelihood
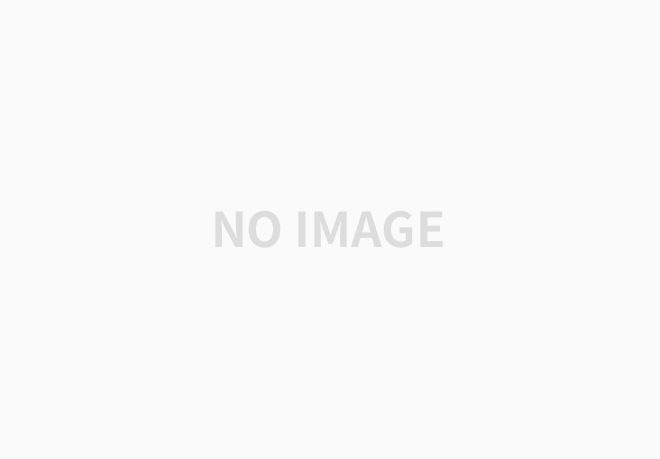
어떤 Dataset X가 고정되어 있을 때,
확률 분포 (distribution = theta) 에 따라 변하는 값.
고정된 X 에서,
현재 확률 분포의 확률값 P(x | theta) 들을 모두 곱한 값을 Likelihood 라고 한다.
이 Likelihood 값이 클수록,
해당 확률 분포(의 파라미터 theta)가
관측된 데이터셋 X를 더욱 잘 설명한다고 해석할 수 있다.
◎ References
< StatQuest, Probability is not Likelihood. Find out why!!! >
< Dlearner의 자기계발 블로그, [기초통계] 확률(Probability) vs 우도(가능도,Likelihood) >
'Data Science' 카테고리의 다른 글
XGBoost for regression (0) | 2023.05.05 |
---|---|
Gradient Boosting (그래디언트 부스팅) for classification (0) | 2023.05.03 |
Linear Regression (0) | 2023.05.01 |
p-value & p-hacking (0) | 2023.04.28 |
Gradient Boosting (그래디언트 부스팅) for regression (0) | 2023.04.28 |